T4P: Test-Time Training of Trajectory Prediction via Masked Autoencoder and Actor-specific Token Memory
Jun 17, 2024ยท
,,,,ยท
0 min read

Daehee Park
Jaeseok Jeong
Sung-Hoon Yoon
Jaewoo Jeong
Kuk-Jin Yoon
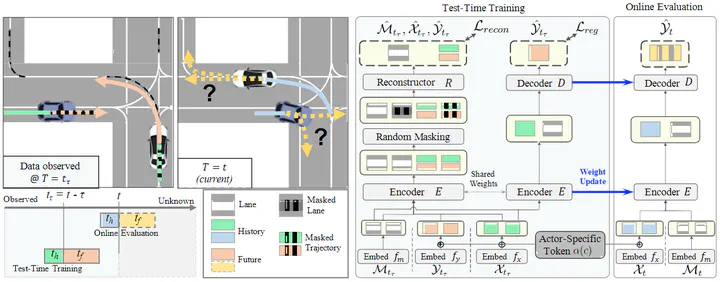
Abstract
Trajectory prediction is a challenging problem that requires considering interactions among multiple actors and the surrounding environment. While data-driven approaches have been used to address this complex problem they suffer from unreliable predictions under distribution shifts during test time. Accordingly several online learning methods have been proposed using regression loss from the ground truth of observed data leveraging the auto-labeling nature of trajectory prediction task. We mainly tackle the following two issues. First previous works underfit and overfit as they only optimize the last layer of motion decoder. To this end we employ the masked autoencoder (MAE) for representation learning to encourage complex interaction modeling in shifted test distribution for updating deeper layers. Second utilizing the sequential nature of driving data we propose an actor-specific token memory that enables the test-time learning of actor-wise motion characteristics. Our proposed method has been validated across various challenging cross-dataset distribution shift scenarios including nuScenes Lyft Waymo and Interaction. Our method surpasses the performance of existing state-of-the-art online learning methods in terms of both prediction accuracy and computational efficiency. The code is available at
.
Type
Publication
CVPR 2024